Show code cell source
# import libraries
import pandas as pd
import numpy as np
import nltk
nltk.download('punkt', quiet=True) # download punkt (if not already downloaded)
from nltk import word_tokenize, sent_tokenize
import spacy
from sklearn.feature_extraction.text import CountVectorizer
from sklearn.preprocessing import OneHotEncoder
import matplotlib.pyplot as plt
import tensorflow as tf
import tensorflow_hub as hub
# change this to your own data directory
data_dir = "data/"
# read and preprocess data
text_file_name = "osdg-community-data-v2023-01-01.csv"
text_df = pd.read_csv(data_dir + text_file_name,sep = "\t", quotechar='"')
col_names = text_df.columns.values[0].split('\t')
text_df[col_names] = text_df[text_df.columns.values[0]].apply(lambda x: pd.Series(str(x).split("\t")))
text_df = text_df.astype({'sdg':int, 'labels_negative': int, 'labels_positive':int, 'agreement': float}, copy=True)
text_df.drop(text_df.columns.values[0], axis=1, inplace=True)
14.8. Solutions to Exercises: Sections 1 to 4#
14.8.1. Preprocessing#
Exercise 1
Answers may vary.
Exercise 2
Answers may vary.
Exercise 3
The following code removes any rows that contain only N/A values. In this case, there are no such rows to remove.
nrows_old = text_df.shape[0]
text_df.dropna(axis=0, how='all', inplace=True)
print("Number of rows removed:", nrows_old - text_df.shape[0])
Number of rows removed: 0
The next line of code checks for the existence of any remaining N/A values. It turns out that there are none.
text_df.isna().any()
doi False
text_id False
text False
sdg False
labels_negative False
labels_positive False
agreement False
dtype: bool
Whether or not entries with N/A values should be removed depends on the dataset and the nature of the problem. Sometimes, entries with N/A values should be dropped, while at other times, they should be kept unchanged, or replaced with interpolated or placeholder values. Consult the pandas
documentation for more information about how to deal with missing values in dataframes.
Exercise 4
After filtering the dataset, we inspect it using the info()
function.
# filter the dataset
text_df = text_df.query("agreement > 0.5 and (labels_positive - labels_negative) > 2")
text_df.reset_index(inplace=True, drop=True)
# inspect it
text_df.info()
<class 'pandas.core.frame.DataFrame'>
RangeIndex: 24669 entries, 0 to 24668
Data columns (total 7 columns):
# Column Non-Null Count Dtype
--- ------ -------------- -----
0 doi 24669 non-null object
1 text_id 24669 non-null object
2 text 24669 non-null object
3 sdg 24669 non-null int64
4 labels_negative 24669 non-null int64
5 labels_positive 24669 non-null int64
6 agreement 24669 non-null float64
dtypes: float64(1), int64(3), object(3)
memory usage: 1.3+ MB
We have 40062 entries with 7 features (see section 1 for details). The data types range from object
(likely denoting strings) to int64
(integers) to float64
(floating-point numbers). This is a reasonable amount of data to work with.
Exercise 5
The Porter and Snowball stemmers are largely comparable, while the Lancaster stemmer is the most aggressive. As a result, the Lancaster stemmer is likely to have the most trouble on a larger set of tokens.
Exercise 6
Answers may vary. Some possible observations include the fact that stemmers tend to remove affixes (such as -ing
, -ed
, and -s
in English) and the fact that irregular words are particularly likely to give the stemmers trouble.
Exercise 7
Answers may vary.
Exercise 8
Answers may vary. Some possible entity labels include GPE
(“nationalities or religious or political groups”), TIME
(“times smaller than a day”), QUANTITY
(“measurements, as of weight or distance”), and WORK_OF_ART
(“titles of books, songs, etc.”).
Exercise 9
Sample code solution:
# load trained pipeline
nlp = spacy.load('en_core_web_sm')
# perform NER on random sample in both original and lower case
sample = text_df['text'].sample(1).values[0]
doc = nlp(sample)
print('ORIGINAL CASE')
spacy.displacy.render(doc, style='ent', jupyter=True)
print('\nLOWERCASE')
doc = nlp(sample.lower())
spacy.displacy.render(doc, style='ent', jupyter=True)
ORIGINAL CASE
LOWERCASE
Answers may vary depending on the samples chosen. This sample demonstrates that the model sometimes confuses organizations with people. Additionally, it shows that the model often fails to recognize organization names (especially abbreviated ones) when they are converted to lowercase.
Exercise 10
Answers may vary.
14.8.2. About Text Data#
Exercise 1
# get document-term matrix
docs = text_df.text
cv = CountVectorizer()
cv_fit = cv.fit_transform(docs)
# get feature names and total counts
feature_names = cv.get_feature_names_out()
total_counts = cv_fit.sum(axis=0)
# get the index of the most frequent word
most_freq_feature = total_counts.argmax()
# get the most frequent word itself
most_freq_token = feature_names[most_freq_feature]
print(f"Most frequent word: '{most_freq_token}'")
Most frequent word: 'the'
Exercise 2
# get document-term matrix with stop words removed
cv2 = CountVectorizer(stop_words='english') # exclude English stop words
cv2_fit = cv2.fit_transform(text_df.text)
original_len = len(cv.vocabulary_) # length of the original vocabulary (with stop words)
new_len = len(cv2.vocabulary_) # length of the new vocabulary (without stop words)
stopwords = cv2.get_stop_words()
print('Length of the original vocabulary (with stop words):', original_len)
print('Length of the new vocabulary (without stop words):', new_len)
print('Number of stop words:', len(stopwords))
print('Difference between original and new vocabularies:', original_len - new_len)
Length of the original vocabulary (with stop words): 45738
Length of the new vocabulary (without stop words): 45440
Number of stop words: 318
Difference between original and new vocabularies: 298
The difference between the original and new vocabularies is less than the number of stop words. This is because not all of the stop words actually occur in the original vocabulary. The following code lists the stop words that are missing from the original vocabulary. Note how the difference between the original and new vocabulary lengths (298) added to the number of missing stopwords (20) is equal to the total number of stop words (318).
missing_stopwords = stopwords - cv.vocabulary_.keys()
print(f'{len(missing_stopwords)} missing stopwords:', missing_stopwords)
20 missing stopwords: {'whereafter', 'whence', 'noone', 'thereupon', 'i', 'thence', 'a', 'latterly', 'yours', 'whereupon', 'couldnt', 'whoever', 'anyhow', 'hasnt', 'whither', 'hers', 'amoungst', 'hereupon', 'yourselves', 'beforehand'}
Exercise 3
# get feature names and total counts
feature_names = cv2.get_feature_names_out()
total_counts = cv2_fit.sum(axis=0)
# get the index of the most frequent word
most_freq_feature = total_counts.argmax()
# get the most frequent word itself
most_freq_token = feature_names[most_freq_feature]
print(f"Most frequent word: '{most_freq_token}'")
Most frequent word: 'countries'
Exercise 4
First, we fit the one-hot encoder to the sample text.
sample_text = text_df.text.iloc[12737].lower()
tokens = nltk.word_tokenize(sample_text)
def ohe_reshape(tokens):
return np.asarray(tokens).reshape(-1,1)
ohe = OneHotEncoder(handle_unknown='ignore') # encode unknown tokens as vectors of all zeros
ohe.fit(ohe_reshape(tokens));
Next, we transform each token only once by using a set to remove duplicates.
token_set = list(set(tokens))
encodings = ohe.transform(ohe_reshape(token_set)).toarray() # encode the tokens
There are multiple ways to check that the resulting encodings are unique, but one simple way is to use the pandas
library. The following code transforms the encodings into a pandas
dataframe and then verifies that there are no duplicates. This confirms that each learned token has a unique encoding.
pd.DataFrame(encodings).duplicated().any()
False
Exercise 5
print('SDG:', text_df.sdg.iloc[118])
print('Text:', text_df.text.iloc[118])
SDG: 5
Text: "Female economic activities were critically examined and new light was shed on existing conceptions of traditional housework. Oxford University Press, 2007). An edited version of Ihe chapter is available al www.rci.rutgers.edu/~cwgl/globalcenler/charlotte/UN-Handbook.pdf. Targets were also set for the improvement of women's access to economic, social and cultural rights, including improvements in health, reproductive services and sanitation. The women in development approach is embodied in article 14 of the Convention, which focuses on rural women and calls on States to ensure that women ""participate in and benefit from rural development"" and also that they ""participate in the elaboration and implementation of development planning at all levels"".15 Participation is an important component of the right to development, as discussed below."
The most frequent words are “women” and “development”, which occur 4 times each. This, together with the label of SDG 5 (gender equality), suggests that this document is about equality for women.
Exercise 6
Each token in a given document, except for the first and last, is grouped into two different bigrams (one with the previous token, and another with the next token). In this case, the large number of distinct bigrams in the entire corpus likely leads to a bigram vocabulary that is larger than the corresponding unigram vocabulary. However, many of the unigrams may occur more often than many of the bigrams do, making the total count of bigrams smaller than the total count of unigrams.
Exercise 7
count_vectorizer = CountVectorizer(ngram_range=(3,3), stop_words='english')
count_vector = count_vectorizer.fit_transform(docs)
print('Total count of trigrams (without stop words):', count_vector.sum())
print('Number of unique trigrams (without stop words):', len(count_vectorizer.vocabulary_))
Total count of trigrams (without stop words): 1301713
Number of unique trigrams (without stop words): 1214215
The total count of trigrams is smaller than the total count of bigrams, but the number of unique trigrams is larger than the total number of unique bigrams. The explanation for this is similar to the reasoning offered in the solution to the previous exercise, but substituting bigrams for unigrams and trigrams for bigrams.
Exercise 8
Answers may vary depending on the sentences chosen.
Exercise 9
tp = 398
fp = 153
fn = 83
precision = tp / (tp + fp)
recall = tp / (tp + fn)
f1 = 2 * (precision * recall)/(precision + recall)
print(f'Precision = {precision}, recall = {recall}, f1 = {f1}')
Precision = 0.7223230490018149, recall = 0.8274428274428275, f1 = 0.7713178294573643
Exercise 10
Answers may vary depending on the parameters chosen. Here is a sample answer using the parameters ngram_range = (2,2)
(for bigrams), stop_words = 'english'
, and min_df = 3
.
docs = text_df.text
count_vectorizer = CountVectorizer(ngram_range=(2,2), stop_words='english', min_df=3)
count_vector = count_vectorizer.fit_transform(docs).toarray()
term_freq = pd.DataFrame({"term": count_vectorizer.get_feature_names_out(), "freq" : count_vector.sum(axis=0)})
# find the frequencies of the 5 most common bigrams
term_freq.sort_values(by='freq', ascending=False).iloc[0:5]
term | freq | |
---|---|---|
27774 | human rights | 1981 |
10071 | climate change | 1301 |
20488 | et al | 1167 |
40775 | oecd countries | 898 |
26527 | health care | 881 |
docs = text_df.text
count_vectorizer = CountVectorizer(ngram_range=(2,2), stop_words='english', min_df=3)
count_vector = count_vectorizer.fit_transform(docs).toarray()
count_vector_df = pd.DataFrame(count_vector, columns=count_vectorizer.get_feature_names_out())
# find the frequencies of the 5 most common bigrams
term_freq.sort_values(by='freq', ascending=False).iloc[0:5]
Exercise 11
def analyze_frequency(corpus, stop_words=None):
# create term-document matrix
count_vectorizer = CountVectorizer(ngram_range=(1,1), stop_words=stop_words)
count_vector = count_vectorizer.fit_transform(corpus).toarray()
# calculate frequencies of 50 most frequent terms
freq_df = pd.DataFrame(
{"term": count_vectorizer.get_feature_names_out(), "freq" : count_vector.sum(axis=0)}
).sort_values(by='freq', ascending=False)
# calculate cumulative word counts
csum = np.cumsum(freq_df.iloc[0:50].freq).values
# create plot
fig, ax = plt.subplots()
plt.plot(csum)
ax.set_ylabel('cumulative word count')
ax.set_xlabel('rank')
ax.set_title('Cumulative Word Count (Most Frequent to 50th Most Frequent)')
# calculate comparison
comp = csum[-1] / freq_df.freq.sum()
return (freq_df.iloc[0:50], ax, f'{comp:.2%}')
Exercise 12
First, we obtain our corpus:
sdg8 = text_df[text_df.sdg == 8].text
Then we run the function from the previous exercise on that corpus and examine the results:
(top_50_words, plot, pct) = analyze_frequency(sdg8)
print('Level of cumulation percentage:', pct)
print()
print('Top 50 words:')
top_50_words
Level of cumulation percentage: 38.58%
Top 50 words:
term | freq | |
---|---|---|
6933 | the | 5400 |
4806 | of | 3294 |
636 | and | 3261 |
3578 | in | 2777 |
7000 | to | 2618 |
3018 | for | 1212 |
3842 | is | 1005 |
6932 | that | 745 |
712 | are | 734 |
4843 | on | 694 |
747 | as | 683 |
7546 | with | 601 |
2498 | employment | 566 |
927 | be | 550 |
1137 | by | 539 |
3994 | labour | 530 |
6958 | this | 485 |
7565 | workers | 430 |
4888 | or | 386 |
7560 | work | 365 |
3326 | have | 360 |
3094 | from | 353 |
4552 | more | 346 |
590 | also | 331 |
3856 | it | 314 |
1771 | countries | 312 |
627 | an | 303 |
4729 | not | 300 |
798 | at | 290 |
3322 | has | 274 |
7513 | which | 273 |
6935 | their | 266 |
6929 | than | 256 |
3883 | job | 248 |
7222 | unemployment | 243 |
1162 | can | 241 |
4317 | market | 235 |
4921 | other | 228 |
7451 | was | 220 |
6201 | sector | 215 |
6946 | these | 213 |
3269 | growth | 212 |
6947 | they | 209 |
6735 | such | 209 |
2396 | economic | 202 |
6451 | social | 199 |
3601 | income | 189 |
7059 | training | 187 |
4804 | oecd | 186 |
939 | been | 184 |
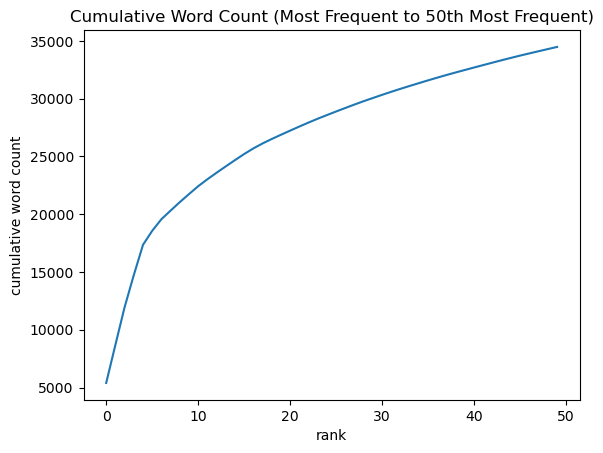
Exercise 13
With stop word removal:
docs = text_df.text
(top_50_words, plot, pct) = analyze_frequency(docs, 'english')
print('Level of cumulation percentage:', pct)
print()
print('Top 50 words:')
top_50_words
Level of cumulation percentage: 12.27%
Top 50 words:
term | freq | |
---|---|---|
10295 | countries | 7761 |
44859 | women | 5984 |
12072 | development | 5312 |
19188 | health | 4685 |
44337 | water | 4664 |
33322 | public | 4591 |
38326 | social | 4538 |
13847 | education | 4535 |
31876 | policy | 4367 |
21846 | international | 4360 |
24043 | law | 4240 |
14495 | energy | 4224 |
27973 | national | 4087 |
35753 | rights | 3905 |
29304 | oecd | 3547 |
13726 | economic | 3517 |
43320 | use | 3391 |
28342 | new | 3337 |
24376 | level | 3267 |
20913 | income | 3171 |
32235 | poverty | 3167 |
11084 | data | 3078 |
8513 | climate | 3033 |
18280 | government | 3012 |
7227 | care | 3002 |
37356 | services | 2999 |
17714 | gender | 2991 |
20001 | human | 2975 |
5194 | based | 2879 |
39986 | support | 2759 |
44896 | work | 2742 |
19483 | high | 2674 |
4016 | areas | 2611 |
37030 | sector | 2583 |
41272 | time | 2581 |
31873 | policies | 2477 |
36770 | school | 2466 |
25497 | management | 2465 |
2098 | access | 2424 |
7882 | change | 2416 |
18543 | growth | 2386 |
19484 | higher | 2367 |
21274 | information | 2363 |
20898 | including | 2357 |
15415 | example | 2318 |
24819 | local | 2285 |
33571 | quality | 2255 |
20724 | important | 2236 |
25028 | low | 2217 |
12270 | different | 2211 |
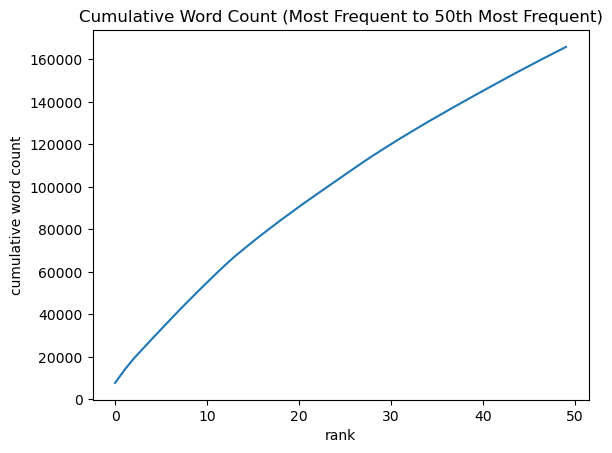
Without stop word removal:
(top_50_words, plot, pct) = analyze_frequency(docs, None)
print('Level of cumulation percentage:', pct)
print()
print('Top 50 words:')
top_50_words
Level of cumulation percentage: 36.81%
Top 50 words:
term | freq | |
---|---|---|
41229 | the | 143100 |
29487 | of | 95834 |
3469 | and | 93357 |
20921 | in | 67152 |
41630 | to | 64701 |
16900 | for | 30010 |
22403 | is | 25175 |
41221 | that | 20395 |
4217 | as | 18926 |
29700 | on | 18365 |
4040 | are | 18215 |
6918 | by | 14171 |
45094 | with | 14162 |
41378 | this | 14153 |
5337 | be | 12608 |
17320 | from | 9833 |
29892 | or | 9382 |
22504 | it | 9364 |
19212 | have | 9070 |
3397 | an | 7885 |
10354 | countries | 7761 |
4447 | at | 7643 |
19159 | has | 7551 |
41248 | their | 7356 |
28988 | not | 7182 |
3182 | also | 7151 |
44893 | which | 6968 |
27490 | more | 6953 |
41327 | these | 6246 |
7121 | can | 6016 |
45152 | women | 5984 |
40001 | such | 5612 |
12135 | development | 5312 |
30114 | other | 5018 |
41211 | than | 4751 |
19298 | health | 4685 |
41339 | they | 4666 |
44606 | water | 4664 |
33516 | public | 4591 |
38540 | social | 4538 |
13916 | education | 4535 |
5660 | between | 4466 |
5401 | been | 4377 |
32070 | policy | 4367 |
21975 | international | 4360 |
44573 | was | 4339 |
24180 | law | 4240 |
14571 | energy | 4224 |
6886 | but | 4088 |
28132 | national | 4087 |
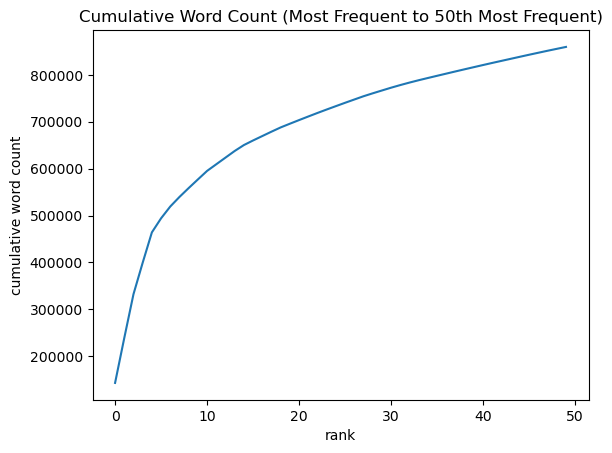
Frequent words that are not stop words, such as ‘countries’, ‘women’, and ‘development’, occur often enough to show up in both lists. As one might expect, stop words such as ‘the’, ‘of’, and ‘and’ occur much more frequently than terms that are not stop words. As a result, the level of cumulation percentage is much smaller and the cumulative word count curve is more linear with stop word removal than without stop word removal.
14.8.3. Document Embedding#
Exercise 1
After creating sentence_df
, we can compare its dimensions to those of text_df
.
def tokenize_into_sentences(corpus):
corpus_sentence = []
corpus_sdg = []
corpus_sample = []
for (text, sdg, i) in iter(zip(corpus.text, corpus.sdg, corpus.index)):
sentences = nltk.sent_tokenize(text)
corpus_sentence += sentences
corpus_sdg += [sdg]*len(sentences)
corpus_sample += [i]*len(sentences)
sentence_df = pd.DataFrame({"text": corpus_sentence, "sdg": corpus_sdg, "sample": corpus_sample})
return sentence_df
sentence_df = tokenize_into_sentences(text_df)
print('text_df dimensions:', text_df.shape)
print('sentence_df dimensions:', sentence_df.shape)
text_df dimensions: (24669, 7)
sentence_df dimensions: (92839, 3)
The dimensions of text_df
represent the number of sample texts and the number of features, respectively. The dimensions of sentence_df
represent the number of sentences and the number of features, respectively.
Exercise 2
Student answers may vary. As a sample answer, we choose a text containing direct quotations, which can increase the difficulty of sentence tokenization:
text_df.text.loc[465]
'When asked “Have you no morals?” Alfred Doolittle in George Bernard Shaw’s Pygmalion answered: “Can’t afford them governor. Neither could you if you was as poor as me.” The modern concept of human rights underpins a moral society and holds governments responsible for fulfilling these rights. From informed consent to the right to privacy civil and political rights have dominated the human rights focus of the HIV-1 epidemic. Yet the economic and social rights of people with HIV-1 infection in particular the rights to health care and to share in scientific advances are glaringly disparate between rich and poor countries. This disparity has become the focus of debate in transnational HIV-1 vaccine research. (excerpt)'
Despite the increased difficulty, the sentence tokenizer is able to separate out the sentences correctly:
sentence_df[sentence_df['sample'] == 465]
text | sdg | sample | |
---|---|---|---|
1785 | When asked “Have you no morals?” Alfred Doolit... | 16 | 465 |
1786 | Neither could you if you was as poor as me.” T... | 16 | 465 |
1787 | From informed consent to the right to privacy ... | 16 | 465 |
1788 | Yet the economic and social rights of people w... | 16 | 465 |
1789 | This disparity has become the focus of debate ... | 16 | 465 |
1790 | (excerpt) | 16 | 465 |
Exercise 3
Answers may vary depending on the samples chosen. For simplicity, this sample solution chooses two samples in text_df
with the same number of sentences.
samples = text_df.loc[[32,6]]
sentences = tokenize_into_sentences(samples)
sentences
text | sdg | sample | |
---|---|---|---|
0 | This points to the possibility that the effect... | 1 | 32 |
1 | One possible explanation for this is that incr... | 1 | 32 |
2 | These results are similar to those obtained by... | 1 | 32 |
3 | This analysis is presented in the following se... | 1 | 32 |
4 | Prescription rates appear to be higher where l... | 8 | 6 |
5 | There is also a possible relationship between ... | 8 | 6 |
6 | This may arise after the definition of disabil... | 8 | 6 |
7 | Krueger (2017(47)) found that around one-fifth... | 8 | 6 |
# change this to your own embedding directory
embedding_dir = "embeddings/"
# load the embedding
embed = hub.load(embedding_dir + "universal-sentence-encoder_4")
sdg1_embedding = embed(sentences[sentences['sdg'] == 1].text.tolist())
sdg8_embedding = embed(sentences[sentences['sdg'] == 8].text.tolist())
np.tensordot(sdg1_embedding, sdg8_embedding)
array(0.2418205, dtype=float32)